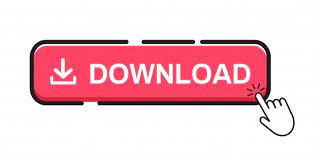
Models investigated were: Poisson (PS), Poisson with Markov elements (PMAK), Poisson with a mixture distribution for individual observations (PMIX), Zero Inflated Poisson (ZIP), Generalized Poisson (GP) and Negative Binomial (NB). Simulations of 100 data sets were performed in NONMEM for each probability distribution with parameter values derived from a real case study on 551 epileptic patients. The aim of this study was to explore the estimation accuracy of population parameters from six count models, using two different methods and programs. There has been little evaluation of maximum likelihood approximation methods for non-linear mixed effects modelling of count data. The Bayesian Markov Chain Monte Carlo method, used in this study for point estimation, performed worst in all tested metrics. FOCE/LAPLACE was the method with the shortest runtime for all models, followed by iterative two-stage. The methods relative robustness differed between models and no method showed clear superior performance.

The method giving the lowest bias and highest precision across models was importance sampling, closely followed by FOCE/LAPLACE and stochastic approximation expectation-maximization. Average estimation time for each algorithm and each model was calculated from the runtimes reported by NM7. Simulations of 100 data sets were used to investigate robustness by comparing final estimates obtained after estimations starting from the true parameter values and initial estimates randomly generated using the CHAIN feature in NM7. Simulations of 500 data sets from each PD model were reanalyzed with the available estimation methods to investigate bias and precision.
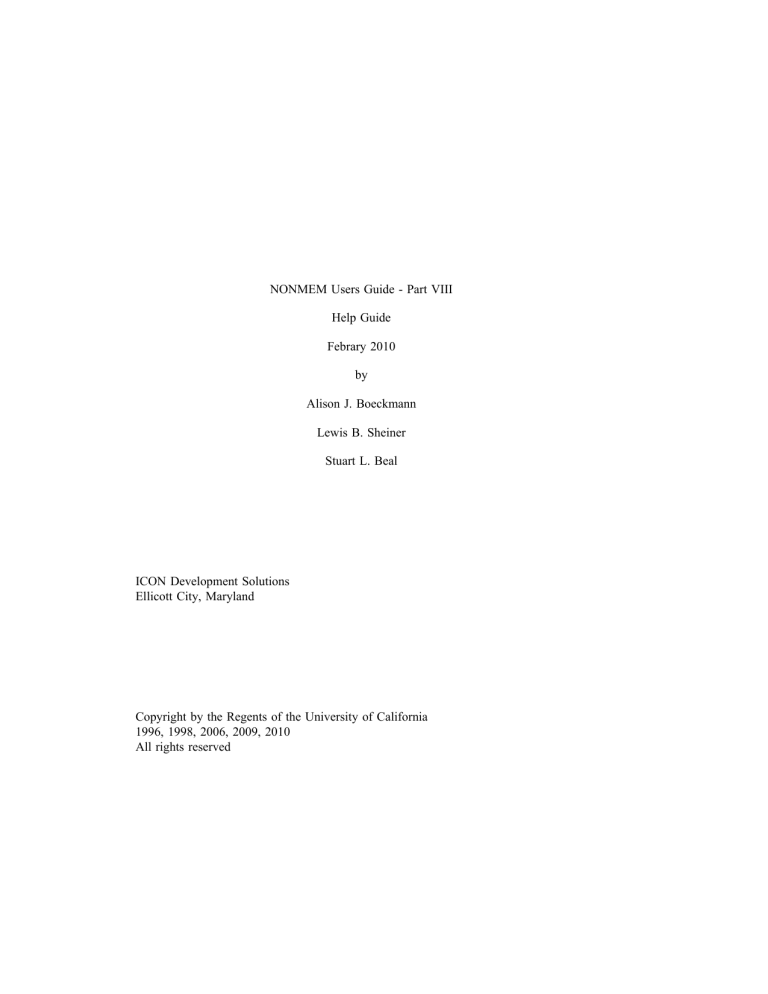
In this study, performance of the estimation methods available in NM7 was investigated with respect to bias, precision, robustness and runtime for a diverse set of PD models. The latest version, NONMEM 7 (NM7), includes several sampling-based estimation methods in addition to the classical methods.
#NONMEM GREATER THAN SOFTWARE#
NONMEM is the most widely used software for population pharmacokinetic (PK)-pharmacodynamic (PD) analyses. The results advocates for the use of bivariate MHMM models when implementation is possible.
#NONMEM GREATER THAN PRO#
The power to detect the drug effect was improved by utilizing a bivariate MHMM model over the univariate MHMM models where the number of subject required for 80% power was 25 with the bivariate MHMM model versus 63 in the univariate MHMM FEV1 model and > 100 in the univariate MHMM PRO model. A drug effect was included on the transition rate probability and the precision of the drug effect parameter improved with increasing magnitude of the parameter.
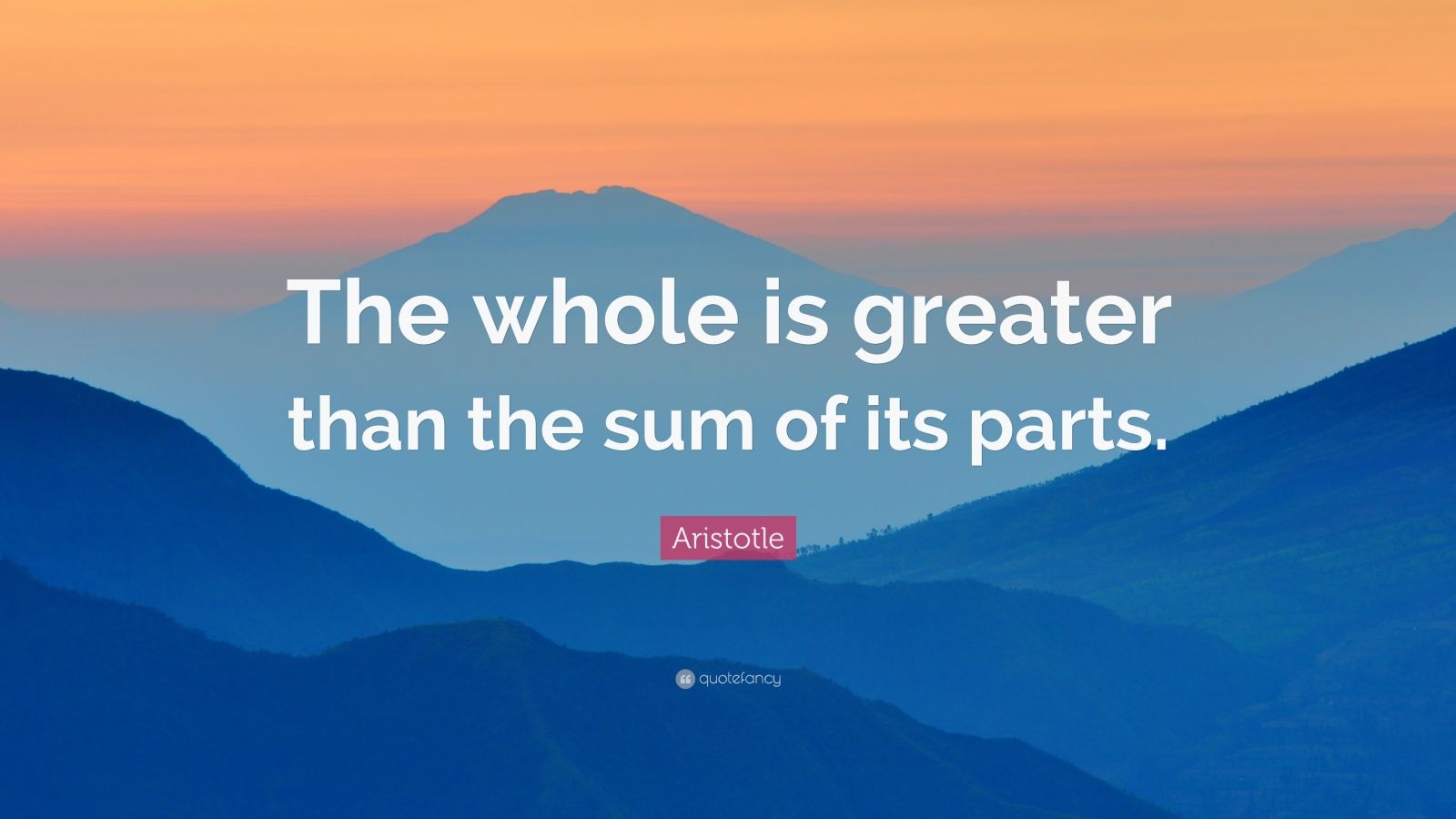
Parameter precision was better with higher magnitudes of the transition probability parameters. Parameter precision was high for all parameters with the exception of the variance of the transition rate dictating the transition from remission to exacerbation (relative root mean squared error > 150%). A bivariate MHMM was developed for simulating and analysing hypothetical COPD data consisting of PRO and FEV1 measurements collected every week for 60 weeks. The influence of including random and covariate effects of varying magnitudes on the parameters in the model was quantified and a power analysis was performed to compare the power of a single bivariate MHMM with two separate univariate MHMMs. Estimation properties in the software NONMEM of model parameters were investigated with and without random and covariate effect parameters. The two hidden states included in the model were remission and exacerbation and two observation sources were considered, patient reported outcomes (PROs) and forced expiratory volume (FEV1).

In this work MHMMs were developed and applied in a chronic obstructive pulmonary disease example. Further, HMMs can be extended to include more than one observation source and are then multivariate HMMs. Adding stochasticity to HMMs results in mixed HMMs (MHMMs) which potentially allow for the characterization of variability in unobservable processes. Hidden Markov models (HMMs) characterize the relationship between observed and hidden variables where the hidden variables can represent an underlying and unmeasurable disease status for example. Non-linear mixed effects models typically deal with stochasticity in observed processes but models accounting for only observed processes may not be the most appropriate for all data.
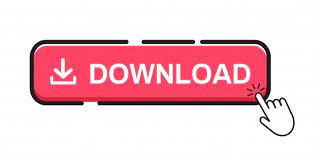